ROBOTICS AND AUTONOMOUS SYSTEMS
Large Language Models Have Pitfalls for National Security
By Laura Heckmann
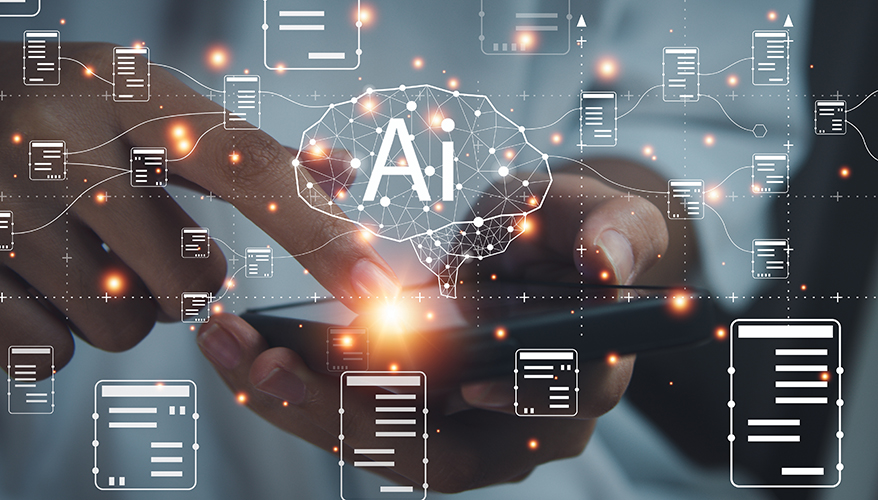
iStock illustration
ORLANDO — Generative artificial intelligence: eerie or exciting? Experts suggest the increasingly intelligent large language models are not something to be feared, but rather tools to make human lives easier, revolutionizing how we learn and train. We just need to learn how to use them effectively.
Critic or proponent, one fact is undeniable: generative AI is here, and it’s getting smarter. Industry and academic experts suggest this leaves one option: get comfortable with it.
Generative AI is an architecture, Svitlana Volkova, chief AI scientist at Aptima Inc., said during a panel discussion at the National Training and Simulation Association’s Interservice/Industry Training, Simulation and Education Conference in November.
“It’s a neural architecture generating pre-trained transformers,” she said. “And there are different versions — models that can learn efficiently from really large data and can be easily adapted to do many tasks.”
Large language models use algorithms and artificial intelligence to mimic human intelligence and generate large sets of data. But it’s only as good as its input, or the learner, which means humans are in the driver’s seat — or can be, if they learn to apply it creatively and responsibly, the panel suggested.
The evolution of the learner has moved through machine learning, supervised learning and deep or representation learning, Volkova said.
Today, it has arrived at what she called in-context learning, “where there is a very large model that has been trained on a lot of data,” she said.
In-context learning is a method of prompt engineering — crafting prompts to feed the model to improve the effectiveness and accuracy of its task. For example, a simple prompt such as “drink water” could be refined to include a plastic cup or filtered water.
Andy Van Schaack, associate professor of the practice of engineering at Vanderbilt University, called prompt engineering “very interesting.
It’s just remarkable how changing a few words can make a big difference.”
New users exploring large language models should not get hung up on the particulars of prompt engineering, he said. “Just launch ChatGPT and start chatting with it. In fact, the first great prompt is, ‘I’m brand new to this, how should I begin?’ And then begin having a conversation with it as though you’re talking to another person.”
Users can take the concept a step further with super prompts — supplying the model with more detailed signals to teach it to respond more effectively.
Keith Brawner, program manager at the Institute for Creative Technologies — a Defense Department-sponsored University Affiliated Research Center working in collaboration with the Army — gave an example of a super prompt: “Hi, my name is Keith. I can read diverse opinions and value them, but those opinions don’t necessarily change my mind. I can talk about the following subjects with expertise. I value complete answers more than I value incomplete answers.”
A super prompt could be pages long, but it can also be “easily automated,” he said. In a way, it already is — by copying and pasting the super prompts before every new chat, he said.
If prompts and super prompts sound overwhelming, Van Schaack said not to worry — they will likely disappear. He speculated the average human will eventually become comfortable enough interacting with AI — and the systems will become sophisticated enough — that prompt engineering will fade into the background.
“I think right now, we do want to have experts who really know how to squeeze out of these systems the very best possible, but the people on the backend … are creating sort of intermediary agents who are translating our ill attempts at prompting into more sophisticated prompts for the system itself,” he said.
At its core, mastering generative AI systems is rooted not in the field of computer science, but human performance, he said.
“There are decades of research on how to get two people to work together productively,” he said. “And so we take those evidence-based approaches for collaborative work and bring it into this world. I think we’re going to see the same positive results.”
But it’s not all positive. Those who fear the rise of the machines are not unwarranted in their concerns, the panel acknowledged. With great power comes great responsibility, and artificial intelligence comes with data poisoning, privacy concerns and security risks.
Van Schaack said there are two main issues with generative AI: privacy and security concerns about the training data and how humans interact with it, and how that data is being used to train models in the future.
He noted the training component for ChatGPT can be turned off, but he simply would not use any commercial systems for confidential or classified work. Concerns over what commercial providers are doing with collected data and who can access it “are absolutely valid,” he said.
From a military training and simulation perspective, when data often is confidential or classified, evaluating trust and privacy depends on what you’re trying to do, Brawner said.
“You see policy coming down … saying, ‘Hey, if large language models help you do your job, please use one of the offline versions. Please don’t let everybody know exactly which reports you are summarizing, but if they help, knock yourself out.’”
Tasks such as providing specific terrain level targeting data, however, are a different story — “Let’s back up a second to this trust and safety and privacy question,” he said. Policy is emerging from the National Institute of Standards and Technology, including certifications of various terrain types for different applications and classified enclaves with classified large language models, he said.
At “the highest levels, when lives are really on the line, all of those questions start to come into play,” he said. The military does have ways to test and verify large language models, such as secure enclaves that ensure large language models are not uploaded, he said.
The size of language models has made security trickier as well, Volkova said. Models used to be much smaller — today, “we’re talking about billions of parameters … sometimes even trillions,” she said. “So, how can we make sure that the terabytes of data [weren’t] poisoned?”
Data poisoning is an adversarial attack that tries to manipulate a model’s training dataset to control its predictive behavior, and it’s a “big issue,” she said. There is no impenetrable guard against it, but Van Schaack said there are measures to mitigate it.
“There are a lot of ways of improving these generative AI systems,” he said. “Faster microprocessors, more memory, write better algorithms, better training datasets, and so the race right now is how do we get these systems that can score better on … these various benchmarks?”
Better quality data could be a start, he said.
Companies are being more thoughtful and deliberate about the data they feed their systems, he said. “So instead of grabbing everything you can on the internet and just dropping every subreddit you can throw on there, they’re being much more careful about it, and they’re also producing guardrails, metaphorically, about how you can respond.”
Volkova said a framework is needed for verifying data and developing proper safeguards and defense mechanisms “to make sure that we are testing these models really carefully.” She said NIST is already working on one, and “policy is moving.”
“We need new technologies to make sure that we’re developing safe, secure and trustworthy generative AI systems, especially with … models that are huge, that it’s practically impossible to validate,” she said. “So, there will be a lot of research in this area, too.”
Throw into the mix of caution and excitement a sense of urgency: a global race to develop the smartest systems is in full swing, Van Schaack said.
“You look at the number of papers that come out, you look at the number of patents that are being produced, there’s as much work being done in the field of AI in China [as] … the United States and certainly other nations,” he said.
The good news is also the bad news: 15 people in an office anywhere can produce “phenomenal models, good or bad,” he said. “And so, it would be naive to think that adversaries … are not capable of beating us in many ways.”
As nations race to develop the smartest systems, another concern could be artificial superintelligence, he said.
“It’s going to develop the next generation of itself that’s going to be even better, and then it just kind of takes off from there,” he said. “And so there is a race to produce that, because intelligence is power.”
Van Schaack predicted five years down the road, computers will be “as capable as we are at any cognitive task,” possibly more, with artificial superintelligence. There will be pockets where a system can’t handle certain types of problems, “but that’s just a PhD thesis away from being solved.”
Humans are already utilizing generative AI to send emails, summarize reports and gather training data. As it continues to rapidly evolve, the focus needs to remain on how humans think about it, Van Schaack said. The human brain will not change.
“Computers are evolving very quickly … the human brain isn’t going to evolve, and so we still need to think about cognitive psychology,” he said. “We still need to think about … psychological principles that help human beings to acquire and retain knowledge and skills, those things won’t change.
“And so, we’ll want to continue to focus on those as we think about training,” he continued. “But how do we use AI to develop those instructional systems? How [will] those systems … adapt based upon the interaction of the learner?” ND
Topics: Robotics